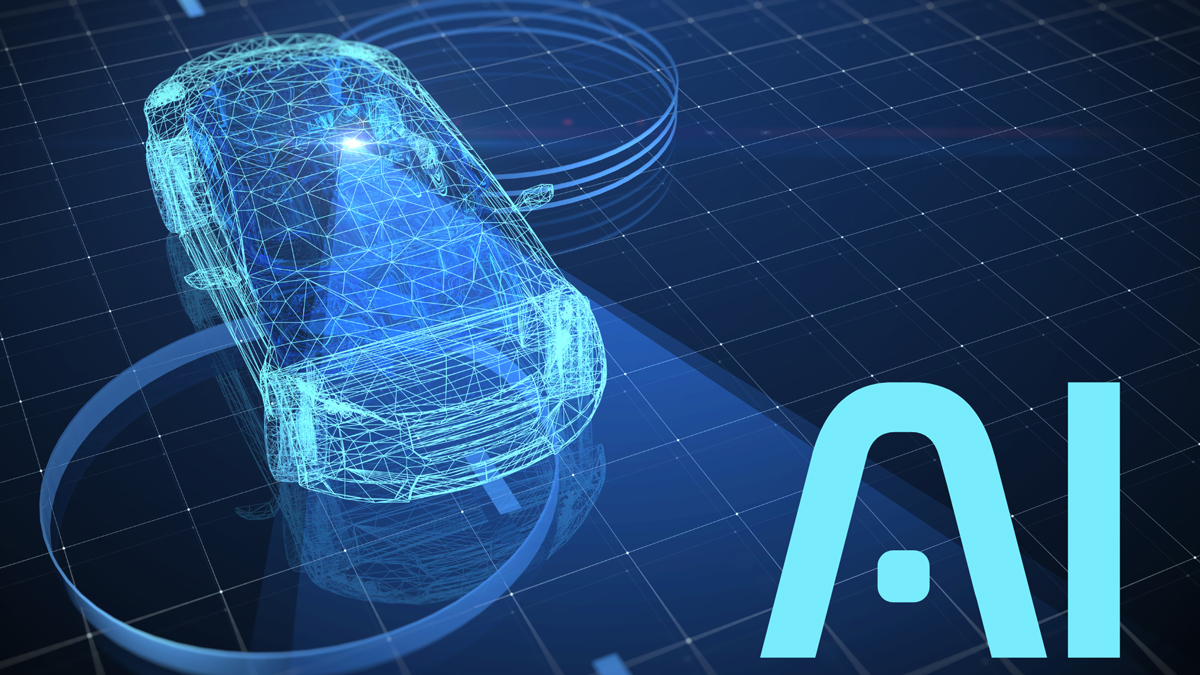
Whenever people talk about the tech transformation, two letters pop up constantly, and rightly so: AI. As ChatGPT, the AI chatbot, put it after a moment’s reflection: ”AI is at the heart of the electric vehicle revolution. As these technologies evolve, we can expect even more innovative solutions that will shape the future of transportation, making it more sustainable, efficient, and enjoyable for everyone.“
The CTI SYMPOSIUM GERMANY in Berlin: Fresh perspectives, new insights
In Berlin, we’ll be shining a light on the hot topic of AI from several different angles. Renowned experts from all fields – from OEMs, suppliers and universities – will share their findings, and discuss how AI is transforming our industry. We’re also dedicating a special Deep Dive session to artificial intelligence, and the topic will feature prominently in two plenary lectures.
In her plenary lecture, Dr Sabine Calvo (VP Engineering Performance, Validations & Digital Transformation, Renault Group) will provide fascinating insights into the challenges of digital transformation from an OEM perspective. In terms of time-to-market acceleration and cost reduction during development, the Renault Group is striving for nothing less than a breakthrough. The solution strategy defines virtual twins for all functions, and ultimately for the complete vehicle, as priority goals. Dr Calvo will speak in detail on generative AI, data power and setting up the requisite backbones, as well as on the tools and methods that have been developed. In future, these will also include immersive simulation tools to involve people in the process.
Software is becoming increasingly important for the customer experience, and hence for customers’ EV purchasing decisions. For OEMs, Software Defined Vehicles (SDVs) are the future. William Wei (CMO & EVP, Skymize) expects this trend to take off in the near future, driven by artificial intelligence in the form of an “AI-First” approach. In his plenary lecture, he will predict deep changes, saying: “The AI-First Paradigm Shift in the Automotive Industry is imminent.” Instead of being mere computers on wheels, vehicles would then become smartphones on wheels, with over-the-air updates and individual features chosen from an app store as the norm. Rather than simply reacting to requirements, vehicles would anticipate passengers’ wishes, and fulfil them individually. We look forward to seeing the picture William Wei paints of this AI-First paradigm shift in his plenary lecture.
Data analysis in modern powertrain development: From concept to SOP and beyond
The technology company AVL has been focusing on data analysis and AI for some time, and both topics now feature strongly in its service portfolio. These innovative technologies are particularly effective during product development in the field of e-mobility. In his presentation, Dr Nikolaus Keuth (AVL) will show how AI and data analysis can help to improve efficiency in the automotive development process. He will examine the challenges European OEMs face, and show how data analysis can help to boost technical efficiency. Dr Keuth will discuss various use cases and toolboxes for data analysis, including BEV fleet monitoring and calibration robustness. Using customer examples, the speaker will show how the AI methods developed and used by AVL to detect anomalies and predict service life can improve product quality, reduce development effort – and even do away with the need for prototypes. And as AVL has already pointed out, the potential cost savings from avoiding warranty claims and recalls are enormous – a further benefit of data analysis that extends beyond the SOP.
Successful deep learning: An impressively accurate virtual temperature sensor
“Sensors play a very important role in determining the performance of our power electronics systems,” says Skander Oueslati (BorgWarner). In his introduction, he will point out the inherent limitations and issues of physical sensors – such as accuracy, price and integration – to explain why BorgWarner is now developing virtual sensors. These are calculation models that can estimate physical values, based on other available information. After development and validation, the virtual sensors will be integrated into the target system as software components, and will replace or support values measured by physical sensors.
The virtual sensor models are being developed with the aid of neural networks and Deep Learning. The training data for the Deep Learning models is generated in a Digital Twin simulation system. In Berlin, Skander Oueslati will present one of the virtual sensor models BorgWarner has developed. For 97% of all predictions, the temperature estimates this sensor delivers are accurate to within 1.6 degrees. That means it can indicate the temperature of the inverter circuit breaker more accurately than the physical sensors currently in use. This suggests that Deep Learning can be used to develop highly accurate virtual sensors, and thus to create lightweight clones of high-precision simulation models that can embedded and run on automotive systems.
Powertrains for heavy-duty electric vehicles: Machine learning accelerates the holistic development process
Unlike component-level optimization of electric motors and transmissions, which is well covered in specialist literature, system-level optimization usually still calls for manual iteration loops. As Anna Rozum (RWTH Aachen University) will explain, this poses particular challenges in the commercial vehicle sector. Here, developers must juggle two opposing factors: a highly cost-conscious development process, and a wide range of limited-production applications. Hence, as the speaker will show, simultaneous system-level optimization aims to accelerate the development process by drastically reducing the number of design iterations (e.g. due to package restrictions). Although genetic algorithms are currently considered state-of-the-art in powertrain optimization, they have a weak point: the time required to identify unfeasible solutions, and – due to the sheer computational intensity involved in cross-component optimization – to reject them completely.
This is where Anna Rozum brings machine learning into play. The solution she presents in Berlin will combine two case studies. First, she will examine differences in algorithm behaviour between vehicle-specific and modular designs. Second, she will compare selected machine learning approaches for holistic optimization in terms of performance, computing time, and suitability for this type of optimization. After presenting the current status of algorithm development, Anna Rozum will round off her talk by discussing possible process improvements.
Mastering complex EV thermal management: Model predictive control and machine learning
The advent of battery-powered electric vehicles (BEVs) has significantly increased the importance of thermal management. In his presentation, Patrick Schutzeich (FEV Europe) will examine current trends in BEV thermal management, and describe the transition from traditional control approaches to model predictive control (MPC) and machine learning (ML).
In BEVs, conventional thermal management methods are often based on heuristic control strategies. But as battery systems grow more complex and environments more variable, there are limits to what these approaches can deliver.
Advanced controls such as MPC and ML, on the other hand, can meet these challenges. For acceptance of EVs to grow further, potential customers expect technical advances in both range and charging times. In simulations, FEV has already demonstrated that MPC-based thermal strategies can help on both counts. As the speaker will make clear, two factors are particularly beneficial: optimized in-car heating, and the avoidance of unnecessary battery cooling. As Patrick Schutzeich‘s presentation will show, we can expect to see important advances from predictively controlled thermal management in future.
Technical drawings: Using AI to harvest a vast treasure trove of data
A considerable amount of valuable data material exists in the form of technical drawings. So far, this data has remained largely unused. As Sascha Ott (Karlsruhe Institute of Technology (KIT) will make clear in Berlin, it’s time to stop extracting information the hard way – meaning manually – and use systematic analysis based on artificial intelligence (AI) instead. In order to extract design-relevant information from technical drawings and convert it into usable knowledge, the speaker proposes a multimodal approach that includes models such as transformer-based large language models (LLM) and neural networks.
Text-based information can be extracted and converted into structured text formats using advanced OCR (Optical Character Recognition). To streamline data processing, various components of technical drawings, such as tables and the actual drawings, are handled separately. The resulting knowledge base should be structured so that AI can use it later on – for example, for functional or error analyses. KIT is planning to demonstrate the approach, possibly in cooperation with potential customers. The demonstration will include possible pre-processing steps, results from the application and their interpretation, and a look at other use cases for the knowledge base generated.
Welcome to the CTI SYMPOSIUM GERMANY 2024
Why click or swipe when you can shake hands instead? Take a break from the digital world – and enjoy relaxed, real-life conversations about deep learning, digital twins, human and artificial intelligence instead. After all, personal contacts with colleagues, speakers and exhibitors and a relaxed atmosphere are what make the CTI SYMPOSIUM GERMANY 2024 so interesting – and so productive.
We look forward to seeing you in Berlin on December 3rd and 4th, 2024!